Nvidia’s position as a leader in artificial intelligence hardware has been pivotal in powering the AI boom. Its cutting-edge GPUs are at the heart of the generative AI revolution, enabling everything from large-scale language models to advanced image synthesis. However, as the demand for AI infrastructure grows exponentially, Nvidia and the industry at large are encountering significant scaling challenges that threaten to slow this unprecedented progress.
The scaling issue is rooted in a mix of supply chain constraints, physical limits of semiconductor technology, and the soaring computational requirements of modern AI models. These challenges, combined with a growing global appetite for AI-powered solutions, pose a complex problem that Nvidia must navigate to sustain its dominance in the AI hardware market.
The Growing Demand for AI Computing Power
The AI boom has led to a dramatic increase in the need for high-performance computing. Language models like OpenAI’s GPT-4, Google DeepMind’s Gemini, and similar systems require billions, sometimes trillions, of parameters to function. Training and deploying these models necessitate massive computational resources, most of which rely on Nvidia’s GPUs.
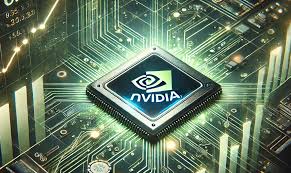
This demand isn’t limited to research labs or tech giants. Businesses across industries are integrating AI to optimize operations, enhance customer interactions, and gain competitive edges. As AI adoption spreads, data centers worldwide are expanding, and Nvidia’s chips are central to this growth. However, this rapid adoption comes at a cost: the infrastructure needed to support AI is struggling to keep pace, and Nvidia’s ability to supply its GPUs in sufficient quantities is being stretched thin.
Bottlenecks in Manufacturing and Supply Chains
Nvidia’s scaling challenges stem partly from the intricate and resource-intensive process of GPU manufacturing. Advanced chips like the H100 or A100 are built on cutting-edge semiconductor nodes, which require significant expertise, capital, and specialized equipment. Taiwan Semiconductor Manufacturing Company (TSMC), Nvidia’s primary supplier, is one of only a few companies globally that can produce these advanced chips. TSMC is operating at near-capacity, and while it continues to expand its facilities, the process is slow and expensive.
The geopolitical landscape adds another layer of complexity. Tensions between Taiwan and China raise concerns about the long-term stability of supply chains, and Nvidia, like many others, is exploring strategies to mitigate this risk. Diversification of manufacturing partners or expanding operations in other regions may help, but such efforts take years to implement fully.
Energy and Cooling Constraints in Data Centers
As AI workloads intensify, so do the energy and cooling requirements of data centers hosting Nvidia’s GPUs. High-density servers running AI models generate significant heat and consume vast amounts of electricity. Many data centers are already reaching their power and cooling thresholds, particularly in regions with older infrastructure or limited energy resources.
Nvidia is working on solutions to address these challenges, such as improving GPU efficiency and promoting liquid cooling technologies. However, retrofitting existing data centers or building new, state-of-the-art facilities is a costly and time-consuming endeavor, creating another obstacle to scaling.
The Role of Software in Alleviating the Scaling Problem
While hardware plays a crucial role, software optimization is equally important in overcoming scaling challenges. Nvidia’s CUDA platform and associated AI libraries are instrumental in maximizing GPU performance. By refining software frameworks and algorithms, Nvidia and its partners can achieve better performance from existing hardware, reducing the immediate need for more GPUs.
Furthermore, innovations in distributed computing allow AI workloads to be spread across multiple GPUs more effectively, increasing overall efficiency. Techniques like model compression, quantization, and sparse computation are also helping to reduce the computational footprint of AI models, though these methods have limitations depending on the application.
Comparing Nvidia’s Position to Industry Rivals
While Nvidia leads the market, competitors like AMD, Intel, and up-and-coming players such as Graphcore and Cerebras are vying for a share of the AI hardware market. Each of these companies offers alternative solutions aimed at addressing scalability. For instance, AMD’s GPUs are gaining traction in cost-sensitive markets, while Intel’s investments in AI accelerators signal a push into specialized hardware.
A comparison highlights Nvidia’s dominance but also underscores the need for diversification:
Company | Strengths | Challenges |
---|---|---|
Nvidia | Market leader, robust software ecosystem | Supply constraints, high costs |
AMD | Competitive pricing, energy-efficient GPUs | Smaller market share, less ecosystem depth |
Intel | AI-specific accelerators, wide hardware base | Lagging in GPU performance |
Graphcore | Innovative AI-specific hardware design | Limited scale and adoption |
Cerebras | Ultra-large-scale AI chips for specific tasks | Narrow focus, high cost |
Future Directions and Potential Solutions
To address the scaling challenges, Nvidia is exploring several strategies. Investing in more advanced manufacturing techniques, such as 2nm technology, could increase chip density and performance, but these innovations are still years away. Partnering with more semiconductor foundries is another possibility, though finding facilities capable of producing high-end GPUs remains a hurdle.
Collaborating with governments and industry leaders to expand domestic chip manufacturing in regions like the U.S. and Europe is a long-term strategy Nvidia and others are pursuing. Initiatives like the CHIPS Act in the U.S. aim to bolster domestic semiconductor production, which could help alleviate supply chain risks.
Meanwhile, Nvidia is also focusing on energy-efficient designs, including the development of lower-power GPUs for specific AI applications. Such innovations could reduce the strain on data centers and help address energy concerns.
Nvidia’s pivotal role in the AI boom comes with immense opportunities and equally significant challenges. Scaling its hardware to meet the growing demand requires a multifaceted approach involving manufacturing, energy efficiency, software optimization, and global collaboration. As the AI revolution continues, Nvidia must navigate these complexities to maintain its leadership and support the technological advancements driving the industry forward. The stakes are high, and how Nvidia addresses these issues will shape the future of AI infrastructure and its own place in this rapidly evolving landscape.
Add Comment